PhD Student Soonbong Lee Wins Two INFORMS Awards for Research on Refugee Resettlement Process
Alongside Professor Vahideh Manshadi and other scholars, Lee created an algorithm that assigns refugees to optimal cities across the U.S. as measured by employment outcomes. Their paper on the subject was honored at the INFORMS Annual Meeting.
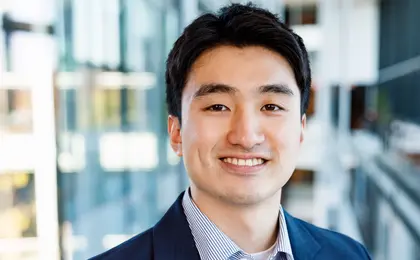
Soonbong Lee, a doctoral student in operations, was honored for his use of operations research to improve the refugee resettlement process at this year’s annual meeting of INFORMS (the Institute for Operations Research and the Management Sciences) in Seattle.
A paper co-authored by Lee, Professor Vahideh Manshadi, and several other scholars, “Dynamic Matching with Post-Allocation Service and its Application to Refugee Resettlement,” won the Michael H. Rothkopf Junior Researcher Paper Prize, which recognizes the best paper on auctions or market design by a young researcher. It also won the annual MSOM Student Paper Competition, which honors an outstanding paper in the field of operations management.
Each year, American resettlement agencies place tens of thousands of refugees in new homes across the country. However, these agencies often struggle to predict where individual refugees are most likely to find employment without stretching local service providers beyond capacity. Lee and Manshadi worked with Kirk Bansak of the University of California, Elisabeth Paulson of Harvard Business School, and Rad Niazadeh of the University of Chicago to create an algorithm that helps agencies make these decisions efficiently and strategically.
The new algorithm uses a machine-learning model to predict the employment outcomes of refugees with certain characteristics (like country of origin, language skills, gender, and age) in different locations. Rather than basing suggestions on outdated information on how many and what kind of refugees are arriving, the algorithm also takes into account the composition of the current year’s refugee pool when making assignments, learning the arrival pattern from week to week and improving its placement suggestions over time. When Lee and his co-researchers tested their algorithm on data from a major U.S. resettlement agency they collaborated with, they found that the algorithm improves employment outcomes and average backlog among local service providers by 50% and 30%, respectively.
Lee, a fourth-year PhD student, came to Yale after earning a B.S. in applied statistics, a bachelor of business administration, and a master’s degree in management science from Yonsei University in Seoul, Korea. He researches the design and operation of online matching platforms, with a particular focus on nonprofit and public-sector applications.