Cleaning Dirty Data
Technologist Amit Garg ’14 and physician Neel Butala ’14 set out to transform population health with machine-learning AI. When they realized that “dirty data” was standing in their way, they pivoted to an AI-based approach to fixing healthcare’s critical data problems. The infrastructure that their company HiLabs is creating could underpin a wide array of advances.

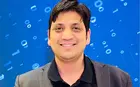
Q: What is the origin of HiLabs?
Neel Butala: Amit and I met as students at Yale SOM. We started thinking about using my medical knowledge and Amit’s technological expertise to work on population health.
Amit Garg: We wanted to use machine learning AI to figure out who’s going to get sick, who’s likely to be re-admitted to the hospital, who needs ongoing special attention for medical conditions. Those are uses where AI really could transform healthcare.
It’s a great idea. We were one of the top business plans in a Yale entrepreneurship competition and got some interest from angel investors. But I remember Dr. Howie Forman brought a healthcare venture capitalist in to talk. The VC said one key thing for entrepreneurs to be successful is flexibility, “Don’t get hung up on ‘I have a great idea.’ Go and test the market. Figure out what customers want and what you can deliver.”
Butala: We quickly got to a point where we could see the healthcare data going into AI models is total garbage.
Garg: We realized that no matter what algorithm we wrote, if the data is not accurate, we were not going to get accurate results.
Q: So you pivoted?
Garg: Currently, healthcare data is siloed in all different kinds of systems with all different kinds of formats and lots of gaps in what is included. It’s understandable, a provider’s job is to deliver care, not to do data entry. Providers are not incentivized to make sure that the data is accurate other than from the reimbursement perspective, so only the data related directly to reimbursement is correct.
Previously, I worked at the Centers for Medicare & Medicaid Services which had lots of initiatives analyzing data in the hopes of providing better care, but it’s very difficult to improve healthcare decision making or health policy given the quality problems in the data.
Running into the same issue as we started HiLabs, we shifted to using machine-learning AI to solve that data quality problem.
Q: Why hasn’t the data quality problem been addressed already?
Butala: Most AI you hear about is plug and play. What we developed is much more advanced—an unsupervised machine-learning AI that is data agnostic. We invested in creating something unique.
Garg: We had a lot of back and forth iterating on the algorithms to get to the point where we are today.
Butala: We figured out how to be really good at cleaning data. Population health is sexy. Being a data infrastructure company sounds very boring. It’s not going to interest the average person. It’s not even going interest the average investor unless they’ve been down in the weeds with the data and understand how hard it is to do what Amit has done. But creating good data infrastructure enables and underpins all the cool things that could be done with clean healthcare data.
Q: Usually infrastructure comes from the public sector. How did you develop this as a business?
Garg: While ultimately this tool is bringing business knowledge and technology together to solve real problems for society, you can’t go to potential customers saying, “OK, I’m solving a problem that will in turn help build another technology.” To be a real-world business we needed to understand who the data-quality problem is currently impacting.
Butala: We started with insurers. When people are shopping for health plans each year during open enrollment, one of the key things they look for is the network of providers. The provider directory is the linchpin for that. Doctors spend an estimated $2.3 billion each year updating provider directories. Each large insurer spends on the order of $100 million updating directories. These are incomprehensibly huge administrative costs.
Garg: In addition to being expensive, the process is manual, so it’s slow and full of errors. On average, it takes health insurers a month to update the data. These are ongoing processes that our AI technology can manage in a more accurate, less costly, and faster way, making the operations of health plans more efficient.
As a result of healthcare reform, most health insurers are competing with each other based on member experience. All our solutions help improve the member experience, directly or indirectly. Signing up for a health plan because you want to see a specific doctor only to find the in-network listing is out of date turns off that member. It’s bad for patients and bad for insurers.
We now have contracts with 3 of the top 10 large health insurers in the country as well as a number of regional health plans. Our platform is analyzing close to one fifth of the insured population of the United States. We have analyzed close to 28 billion records.
Butala: Improving provider directories helps insurers accurately represent the breadth and depth of their networks. It reduces surprise billing because people aren’t relying on inaccurate directories. Further, accurate information about networks helps regulators decide whether health plans are offering the right amount of network adequacy in a given location.
Q: What are the broader impacts of the tools you have developed?
Butala: Cleaning clinical data is another use of our platform. People who have diabetes or high blood pressure or any number of ongoing medical conditions often need additional care associated with those conditions. Sharing data can allow for programs that deliver better care more cost effectively. Value-based care similarly depends on sharing data about patient care. All of those potential improvements in patient care depend on clinical data—patient records.
Just as with provider directories, there are really high error rates in the clinical data. Our platform allows one of our large clients to do data checks that help them clean up all the clinical data that doctors and patients generate that’s really dirty.
Garg: As we solve this data quality problem using smart technology, as we develop data-ingestion processes that are more intelligent and efficient, we’re moving toward the data infrastructure that can take advantage of AI and machine learning. We are creating the ecosystem where adoption of AI within healthcare becomes possible.
Q: Looking ahead, what are HiLabs’ priorities for the next five years?
Garg: We aim to expand our offerings for insurers to make lives easier for providers as well.
Butala: As a doctor, it makes my life easier to not spend time documenting. I can focus on patients if AI is cleaning the medical records, formatting them, and sending everything where it needs to go.
Garg: At some point the tools could also be used in other areas of healthcare such as genomic data, pharmacy data, or clinical trial data. That’s probably the next five years.
Q: This company started when you met at Yale SOM. Why did you each choose to get an MBA? And why Yale SOM as the place to get it?
Garg: I’m an engineer. All I ever wanted to do was create things. I never thought of doing business school until I realized I wanted to create a business and products that could be commercialized. Then I understood I needed the concentrated, structured knowledge that would let me start and lead a company—hiring, organizational culture, finance, strategy, and competition.
When I was looking at different business schools, I was drawn to the specialized healthcare track at Yale SOM. I also saw that Yale SOM is very much embedded within Yale. That was very attractive. I took classes in other schools and attended talks at the medical school and at the law school. Yale SOM was a perfect fit for me.
Butala: I’m fundamentally a physician. I like working to make patients’ lives better. But working one-on-one you can only help a limited number of people. I went to business school to get a perspective on how to affect change in the healthcare system.
I may be biased, but I don’t think there’s a more business-and-society sector than healthcare, so Yale SOM’s focus on business and society was super appealing. And it’s not just words; the mission shapes the focus of classes and impacts the kind of people who are drawn to the school. Yale SOM selects for people who are more well-rounded, have more unique experiences. A lot of people want to run a nonprofit or create mission-driven businesses. Being around people with the mindset of working to improve people’s lives was huge. It created an opportunity to learn from fellow students as much as from faculty.