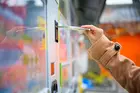
How We Buy When Time Is Short
An experiment with vending machines on train platforms demonstrates the way in which time pressure affects our decision-making—and how marketers and data scientists might use this to their advantage.
Rarely do we shop without distractions. While we’re browsing for some goods we talk on the phone or manage kids or fret about getting a parking ticket or consider what to make for dinner. The fact is, the decisions we make as consumers are guided to a great extent by the invisible contexts in which we make those decisions.
“A large number of behavioral studies in marketing find that consumers tend to behave differently under contextual factors such as time and social pressures,” writes Yale SOM associate professor Kosuke Uetake in a forthcoming article for Management Science. And yet these context factors have remained, for the most part, unexamined in the real world (or outside the lab). “This paper aims at filling the gap by studying the mechanism behind the effect of recommendations under contextual factors, and by investigating how to optimize marketing interventions [based on] contextual factors.” Specifically, Uetake and his coauthors, Kohei Kawaguchi from Hong Kong University of Science and Technology, and Yasutora Watanabe from University of Tokyo, examine the ideal marketing interventions in the face of customer time constraints.
At the heart of the work is data from roughly 32,000 customers of 460 vending machines on train platforms around Tokyo. The machines are embedded with cameras that recognize consumers’ age and gender; the machines make product recommendations based on this information. Between July 15 and July 26, the company that manages the machines rolled out an experiment in which different types and numbers of products were randomly recommended to consumers. A control group received no recommendations.
Uetake and his coauthors then matched consumer choices against train schedules with the idea “that consumers feel more time pressure when the next train is approaching,” Uetake writes. (Trains in Tokyo, they are careful to note, operate punctually.) With these two sets of information, the researchers constructed a model to articulate how time pressure and product recommendations interact to influence purchase decisions.
Most fundamentally, the results demonstrate that product recommendations—to a point—increase consumer attention and boost total vending machine sales by 3.2%. (These effects are weaker with women and 30- to 50-year-olds.) When consumers are under time pressure, however, they pay less attention to recommendations, which “suggests that consumers do not want to be told what to buy when they are in a hurry,” the authors write.
Under time pressure—when a train is approaching—the vending machines would ideally provide fewer recommendations to consumers.
Integrating these two results, the researchers find striking value in a flexible recommendation system: taking time pressure into account increases sales by about 1% compared to simply optimizing recommendations based on demographics. “We find that the contextual policy outperforms the traditional attribute-based recommendation policy,” the researchers write. The increase in sales, in fact, is comparable to the sales increase gained from a 10% discount; but there is no concomitant dip in revenue. As they write, “the context-based recommendation increases the revenue more than a price cut at the margin.”
Ultimately, while this investigation maintains a narrow focus—vending machine purchases on a train platform under time pressure—it has important implications for how data scientists might approach analyses and experimentation design. Many organizations are already adept at generating insights based on customer attributes and even existing behavioral data, and a subset may even do a good job of using that information to generate hypotheses for in-market experimentation. But generating experiments to optimize revenue based on how consumers may be feeling in a particular context is often not considered. “Despite its practical importance,” Uetake and his coauthors write, “how context-based marketing works, and how managers can optimize it are not well understood.”